Creating Predictive AI Models in Data Cloud: Best Practices
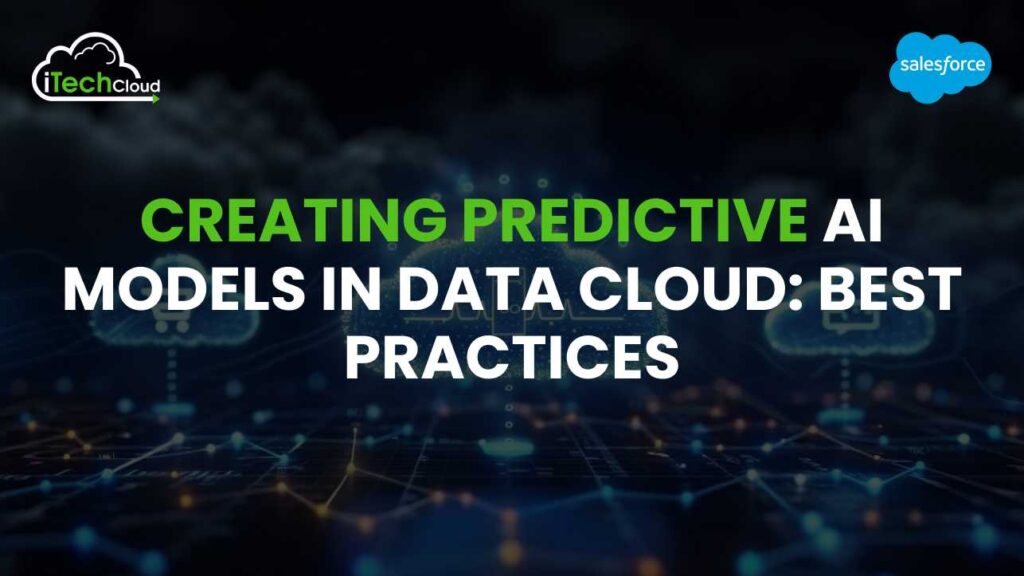
Predictive AI Models in Data Cloud have become a critical asset for businesses looking to leverage data for strategic decision-making. In Salesforce Data Cloud, these models are essential for predicting customer behavior, optimizing marketing strategies, and improving sales forecasting. This blog will cover best practices for creating predictive AI Models in Data Cloud, offering insights into data preparation, model selection, implementation, and monitoring.
Table of Contents
Understanding Predictive AI Models in Data Cloud
Salesforce Data Cloud is a powerful platform that integrates data from various sources to provide a unified view of customers. It allows businesses to leverage AI and machine learning (ML) to create predictive models that can forecast outcomes based on historical data. These models can predict anything from customer churn and purchase likelihood to personalized product recommendations.
Best Practices for Creating Predictive AI Models in Data Cloud
1. Data Preparation
Data preparation is the foundation of any predictive model. The accuracy and reliability of your AI Models in Data Cloud depend heavily on the quality of data fed into it. Follow these steps for effective data preparation:
- Data Cleaning: Remove duplicates, correct errors, and handle missing data to ensure the dataset is clean and reliable.
- Feature Engineering: Transform raw data into features that can improve the model’s predictive power. This may involve creating new variables or transforming existing ones.
- Data Normalization: Standardize data to ensure consistency, especially when integrating data from multiple sources.
- Data Segmentation: Divide the dataset into training, validation, and test sets to evaluate the model’s performance effectively.
2. Model Selection
Choosing the right model is crucial for accurate predictions. AI Models in Data Cloud offers various AI and ML models, each suited to different types of predictions:
- Regression Models: Best for predicting continuous variables such as sales revenue or customer lifetime value.
- Classification Models: Ideal for categorizing data, such as predicting whether a customer will churn.
- Clustering Models: Useful for segmenting customers into distinct groups based on behavior or preferences.
- Time-Series Models: Best for forecasting trends over time, such as monthly sales projections.
Consider the nature of your data and the prediction goals when selecting the model. Utilize Salesforce’s Einstein Prediction Builder for simplified model creation, or delve into more complex models using custom ML algorithms.
3. Training the Model
Training the model involves feeding it with data so it can learn patterns and make predictions. Follow these best practices during the training phase:
- Hyperparameter Tuning: Adjust the model’s parameters to improve performance. This can significantly impact the accuracy of predictions.
- Cross-Validation: Use cross-validation techniques to prevent overfitting and ensure that the model generalizes well to new data.
- Iterative Refinement: Continuously refine the model by retraining it with updated data or tweaking features and parameters.
4. Model Evaluation
Once the model is trained, it must be rigorously evaluated to ensure its accuracy and reliability. Salesforce Data Cloud provides tools for model evaluation, but you should also incorporate the following:
- Confusion Matrix: For classification models, a confusion matrix helps assess the model’s accuracy by showing the true positives, false positives, true negatives, and false negatives.
- ROC-AUC Curve: This curve helps evaluate the performance of classification models, indicating the trade-off between sensitivity and specificity.
- Mean Absolute Error (MAE): For regression models, MAE measures the average magnitude of errors in predictions, providing insight into model accuracy.
- Precision and Recall: For imbalanced datasets, these metrics help evaluate how well the model predicts the minority class.
5. Implementation
After evaluating the model, the next step is implementation. This involves integrating the model into your Salesforce environment where it can start making predictions in real-time. Follow these best practices:
- APIs Integration: Use Salesforce’s APIs to integrate predictive models into your workflows. This allows the model to make predictions on live data.
- Automation: Automate the prediction process by setting up triggers and workflows that initiate model predictions at the right time, such as when a new lead is created.
- User Training: Ensure that end-users understand how to interpret model predictions and incorporate them into decision-making processes.
6. Monitoring and Maintenance
Even after deployment, continuous monitoring and maintenance are crucial to ensure the model remains accurate and relevant.
- Model Drift Detection: Over time, the accuracy of a model can degrade as data patterns change. Regularly monitor for model drift and retrain the model as needed.
- Performance Monitoring: Track key performance indicators (KPIs) related to the model’s accuracy, precision, and recall to ensure it continues to meet business goals.
- Feedback Loop: Implement a feedback loop where the model’s predictions are reviewed, and incorrect predictions are fed back into the model for retraining.
- Scalability: Ensure that the model can handle increased data volume and complexity as your business grows.
7. Ethical Considerations
Incorporating AI and ML into business operations brings up ethical considerations, particularly around data privacy and bias:
- Bias Mitigation: Regularly evaluate the model for bias, particularly in data that might inadvertently disadvantage certain groups. Techniques like re-weighting or adversarial debiasing can help reduce bias.
- Data Privacy: Ensure that the data used for training and predictions complies with relevant data privacy regulations like GDPR. Salesforce Data Cloud provides tools to manage consent and anonymize data.
- Transparency: Make sure that users and stakeholders understand how the model works and how predictions are made. This builds trust and facilitates better decision-making.
Use Cases of Predictive AI Models in Data Cloud
Predictive AI models in Data Cloud harness advanced machine learning and analytics to provide actionable insights and drive business strategies. These models use historical and real-time data to forecast future outcomes, optimize processes, and enhance decision-making. Here’s a detailed overview of their key use cases:
1. Sales Forecasting and Lead Scoring
Sales Forecasting: Predictive AI Models in Data Cloud analyze historical sales data to forecast future sales trends. By examining patterns and correlations between various factors (such as seasonality, market conditions, and customer behavior), these models can predict future sales performance. This enables sales teams to set realistic targets, allocate resources effectively, and plan strategies to meet revenue goals.
Lead Scoring: AI Models in Data Cloud assess the likelihood of a lead converting into a customer by evaluating attributes like engagement level, company size, and past interactions. This scoring helps sales teams prioritize high-potential leads and tailor their approach to increase conversion rates. By focusing on leads with higher scores, businesses can enhance their sales efficiency and effectiveness.
2. Customer Churn Prediction
Predictive models identify signs that a customer might be at risk of leaving. By analyzing engagement metrics, purchase history, and service interactions, these models can pinpoint customers showing potential dissatisfaction or reduced interaction levels. Businesses can then implement targeted retention strategies, such as personalized offers or proactive customer support, to address issues before they lead to churn. This helps in improving customer loyalty and reducing turnover rates.
3. Personalized Marketing Campaigns
Customer Segmentation: AI Models in Data Cloud analyze customer data to segment audiences based on behavior, preferences, and demographics. This segmentation facilitates the development of highly focused marketing campaigns that effectively engage particular customer segments.
Campaign Optimization: Predictive models help in crafting personalized marketing messages and offers. By understanding individual customer preferences and predicting future behaviors, businesses can deliver tailored content, recommend relevant products, and design campaigns that drive higher engagement and conversion rates. This personalization increases the effectiveness of marketing efforts and maximizes return on investment.
4. Optimizing Customer Service
Proactive Support: Predictive AI Models in Data Cloud enhance customer service by forecasting customer needs and potential issues. By analyzing historical service data and customer feedback, these models can suggest optimal solutions and anticipate future service requirements. This allows customer service teams to provide proactive support, reduce response times, and improve customer satisfaction.
Automated Responses: AI can automate routine service interactions by predicting common customer inquiries and providing instant responses. This reduces the burden on service teams and ensures that customers receive timely assistance.
5. Fraud Detection and Prevention
In industries like finance and e-commerce, predictive models are crucial for detecting fraudulent activities. AI analyzes transaction patterns, customer behavior, and historical fraud data to identify anomalies that may indicate fraud. This early detection helps in mitigating risks, preventing financial losses, and ensuring regulatory compliance.
6. Inventory Management and Demand Forecasting
Demand Forecasting: Predictive models analyze historical sales data, market trends, and seasonal factors to forecast demand for products. Accurate demand forecasting helps businesses optimize inventory levels, reducing both excess stock and stockouts.
Inventory Optimization: By predicting future demand, businesses can make informed decisions about purchasing and production. This leads to more efficient inventory management, cost savings, and better alignment with market demand.
7. Employee Productivity and Retention
Performance Prediction: AI Models in Data Cloud assess employee performance by analyzing data such as work patterns, project outcomes, and feedback. This helps in identifying high-performing employees and those who may need additional support or development.
Retention Strategies: Predictive models can also forecast potential retention issues by analyzing factors like job satisfaction, career progression, and engagement levels. HR departments can use these insights to implement targeted interventions, such as career development programs or personalized incentives, to improve employee satisfaction and retention.
8. Product Development and Innovation
Trend Analysis: Predictive models analyze customer feedback, market trends, and competitive data to guide product development. By identifying emerging trends and unmet customer needs, businesses can innovate effectively and stay competitive in the market.
Idea Validation: AI Models in Data Cloud help in assessing the potential success of new product ideas by predicting customer acceptance and market performance. This reduces the risk associated with product development and ensures that resources are invested in viable opportunities.
9. Risk Management and Compliance
Risk Identification: Predictive models assess data from various sources to identify potential risks related to financial operations, legal compliance, and business processes. This proactive approach helps businesses anticipate and mitigate risks before they escalate.
Compliance Monitoring: AI Models in Data Cloud can track and analyze regulatory requirements, ensuring that business practices comply with industry standards and legal regulations. This helps in avoiding compliance issues and maintaining a strong reputation.
Conclusion:
AI models in Data Cloud are transformative tools that drive strategic decision-making and operational efficiency. By leveraging machine learning and advanced analytics, these models enhance sales forecasting, optimize lead scoring, and improve customer retention through predictive insights. They enable highly personalized marketing campaigns and proactive customer service, which can lead to increased engagement and satisfaction.
AI Models in Data Cloud play a crucial role in fraud detection, inventory management, and demand forecasting, helping businesses mitigate risks and manage resources effectively. They also contribute to employee productivity and retention by predicting performance and addressing potential issues before they escalate.
Moreover, AI supports product development by identifying trends and validating new ideas, while also ensuring risk management and regulatory compliance. Overall, AI models in Salesforce Data Cloud empower businesses with actionable insights that drive growth, improve efficiency, and enhance customer and employee experiences.