The Importance of Data Quality in Salesforce
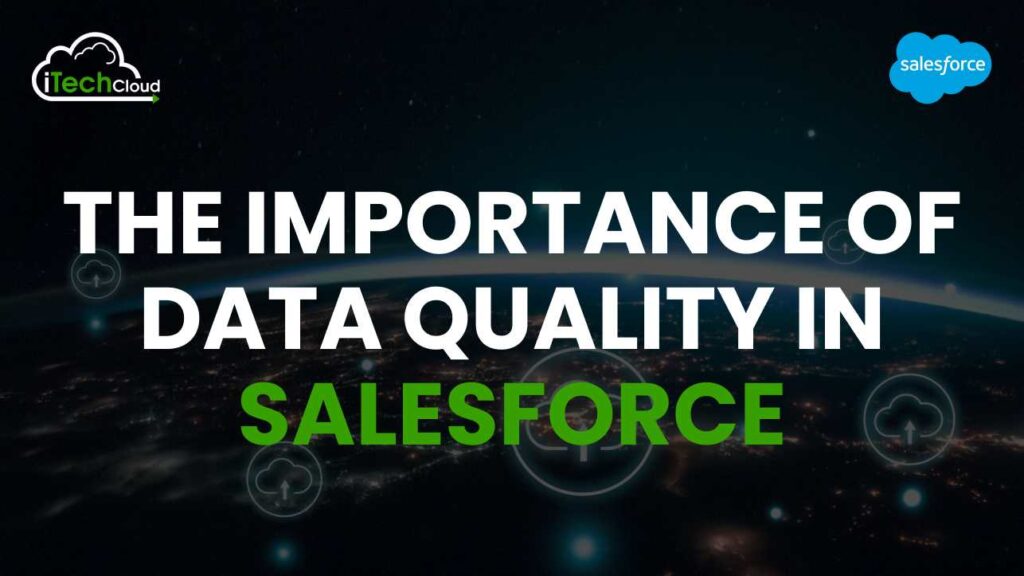
Salesforce is renowned as a leading CRM platform that empowers organizations to manage customer relationships efficiently. Central to its functionality is the management of data, which serves as the lifeblood of Salesforce operations. This data encompasses customer information, sales transactions, marketing interactions, and more, all critical for driving business decisions and customer engagement.
Understanding Data Quality in Salesforce
Data quality in Salesforce refers to the accuracy, completeness, consistency, and relevance of data stored in the platform. It ensures that information is reliable for decision-making and operational use. Key aspects include regular data cleansing, validation rules, and automated processes to maintain consistency. High-quality data enhances user trust, improves reporting accuracy, and supports effective CRM functionalities like segmentation and personalization. Regular audits and data governance strategies are crucial for sustaining data quality over time.
Table of Contents
Key Components of Data Quality in Salesforce
Ensuring high-quality data in Salesforce involves several key components that collectively contribute to accurate, reliable, and useful information management. Here’s a detailed overview:
1. Accuracy:
Data accuracy is fundamental. It means that information stored in Salesforce must be correct and free from errors. This includes ensuring that data entries are entered correctly at the point of capture and that updates or changes are accurately reflected throughout the system. Accuracy ensures that decisions made based on Salesforce data are based on reliable information.
2. Completeness:
Data completeness ensures that all necessary fields and elements within Salesforce records are adequately filled out. This prevents gaps or missing pieces of information that can hinder comprehensive analysis or lead to incomplete customer profiles. Complete data allows for a more holistic view of customers, transactions, or any other entity being managed within Salesforce.
3. Consistency:
Consistency in data ensures uniformity across all records and fields within Salesforce. This includes maintaining standardized formats, units of measure, and values. Consistency is crucial for accurate reporting, effective data analysis, and seamless integration between different Salesforce modules or external systems. It helps in avoiding confusion or errors that may arise from variations in data formatting or representation.
4. Validity:
Validity refers to the adherence of data to defined rules and constraints. It ensures that data values are within acceptable ranges and comply with predefined formats or criteria. For example, valid email addresses, phone numbers with correct formats, or dates that fall within specified ranges. Valid data is essential for maintaining data integrity and ensuring that information stored in Salesforce is meaningful and usable.
5. Timeliness:
Timeliness of data ensures that information is current and reflects the most recent updates or changes. It involves timely updates and synchronization of data across Salesforce and other integrated systems. Timely data enables organizations to make informed decisions based on up-to-date information and reduces reliance on outdated or obsolete data.
6. Integrity:
Data integrity ensures the accuracy, consistency, and reliability of data throughout its lifecycle within Salesforce. It involves maintaining the relationships and dependencies between data elements, ensuring that there are no contradictions or discrepancies within the data. Data integrity measures help in preserving the trustworthiness and reliability of Salesforce data, supporting accurate reporting, and facilitating effective data-driven decision-making.
7. Deduplication:
Deduplication is the process of identifying and removing duplicate records within Salesforce. Duplicate records can arise from various sources, such as data imports, manual data entry errors, or system integrations. Removing duplicates ensures that each record represents a unique entity, reducing confusion and streamlining data management processes. It helps in maintaining a clean and organized Salesforce database, improving user efficiency and data accuracy.
8. Security:
Data security is critical for protecting Salesforce data from unauthorized access, modifications, or deletions. It involves implementing robust security measures, such as user authentication, access controls, encryption, and data masking, to safeguard sensitive information. Secure data management practices ensure compliance with regulatory requirements and protect Salesforce data from potential threats or breaches, preserving data confidentiality, integrity, and availability.
Benefits of High Data Quality in Salesforce
Data quality in Salesforce is foundational to maximizing the potential of Salesforce and reaping numerous organizational benefits across various facets of business operations. From accurate reporting to enhanced customer relationships and efficient operations, here’s a comprehensive exploration of why high data quality matters in Salesforce.
1. Accurate Reporting and Analytics:
At the core of Salesforce’s value proposition lies its ability to generate insightful reports and analytics. However, the reliability of these insights hinges on the quality of underlying data. High-quality data ensures that reports are based on accurate, up-to-date information, providing stakeholders with a clear and reliable view of business performance. This accuracy empowers decision-makers to formulate strategies confidently, leveraging data-driven insights to capitalize on opportunities and mitigate risks effectively.
2. User Adoption:
User adoption is critical to realizing the full potential of Salesforce across an organization. When users trust the data within Salesforce, they are more inclined to engage with the platform consistently. High Data quality in Salesforce fosters user confidence by ensuring that information is reliable and actionable. This, in turn, promotes widespread adoption of Salesforce functionalities, driving productivity and collaboration among teams.
3. Improved Customer Relationships:
In today’s customer-centric landscape, personalized interactions are paramount to fostering loyalty and satisfaction. High-quality data in Salesforce enables organizations to gain a comprehensive understanding of their customers. Accurate customer profiles facilitate tailored communication, personalized offers, and timely responses to inquiries or issues. By delivering superior customer experiences based on reliable data, organizations can strengthen relationships, increase retention rates, and ultimately drive growth.
4. Efficient Operations:
Clean and reliable data streamlines operational processes within Salesforce. It reduces errors and redundancies in data entry, updates, and workflow automation. This operational efficiency not only saves time but also optimizes resource allocation and enhances overall productivity. By eliminating the inefficiencies associated with poor Data quality in Salesforce, organizations can focus their efforts on strategic initiatives and value-adding activities.
5. Cost Savings:
Maintaining high data quality in Salesforce contributes to significant cost savings over time. Organizations incur fewer expenses related to data cleansing, duplicate management, and rectifying errors caused by inaccurate or outdated information. By investing in data quality upfront, businesses can mitigate the financial risks associated with poor data practices and allocate resources more effectively towards innovation and growth initiatives.
6. Compliance and Security:
High-quality data in Salesforce ensures that organizations maintain compliance by adhering to data governance standards and safeguarding sensitive information. By reducing the risks associated with data inaccuracies or breaches, businesses can enhance trust with stakeholders and mitigate potential legal and reputational consequences.
7. Effective Marketing Campaigns:
Marketing effectiveness hinges on the ability to deliver targeted and relevant messages to the right audience. Clean and accurate data enables sophisticated segmentation and personalization within Salesforce. By understanding customer preferences, behavior patterns, and purchase history, organizations can tailor marketing campaigns to resonate with their audience effectively. This targeted approach not only improves campaign ROI but also enhances customer engagement and loyalty over time.
8. Predictive and Prescriptive Insights:
High-quality data fuels advanced analytics capabilities within Salesforce, enabling organizations to derive predictive and prescriptive insights. By analyzing historical data trends and patterns, businesses can forecast future outcomes, identify emerging opportunities, and proactively address challenges. These data-driven insights empower decision-makers to make informed strategic decisions, optimize resource allocation, and gain a competitive edge in the marketplace.
Challenges in Maintaining Data Quality
Maintaining high data quality in Salesforce poses several challenges that organizations must address to ensure the reliability and usefulness of their data:
1. Data Accuracy:
One of the primary challenges is ensuring the accuracy of data entered into Salesforce. Inaccurate data can stem from human error during data entry, outdated information that hasn’t been updated, or integration issues when importing data from external sources. Addressing accuracy requires robust validation rules, regular data audits, and user training to emphasize the importance of entering correct information.
2. Data Completeness:
Ensuring that all necessary data fields are populated is another challenge. Incomplete data can hinder reporting accuracy and limit the effectiveness of Salesforce features like automation and analytics. Organizations need to implement data completeness checks, automate data population where possible, and establish protocols for data validation during entry to mitigate this challenge.
3. Data Consistency:
Consistency refers to ensuring that data is uniform across all records and adheres to established standards. In Salesforce, inconsistencies can arise from varying data entry practices, different interpretations of data fields, or discrepancies between integrated systems. Establishing data governance policies, standardized data entry procedures, and regular data cleansing activities are essential to maintaining consistency.
4. Data Duplication:
Duplicate records are a common issue that can lead to confusion, wasted resources, and inaccurate reporting. Salesforce users may inadvertently create duplicate entries, especially in large organizations with decentralized data entry processes. Implementing duplicate management tools, training users on data deduplication best practices, and periodically conducting data deduplication campaigns are crucial steps to mitigate this challenge.
5. Data Integrity:
Data integrity ensures that data remains accurate and consistent throughout its lifecycle within Salesforce. It involves maintaining referential integrity (relationships between related records), ensuring data is not corrupted or altered unintentionally, and protecting data from unauthorized access or manipulation. Organizations can enhance data integrity by implementing robust security measures, role-based access controls, and regular data integrity checks.
6. Data Timeliness:
Timeliness refers to the freshness and relevance of data within Salesforce. Outdated or stale information can compromise decision-making processes and hinder customer interactions. Ensuring data timeliness involves implementing real-time data synchronization where feasible, establishing data refresh schedules for integrated systems, and enforcing timely updates through user notifications and automated reminders.
7. Data Governance:
Effective data governance is essential for maintaining overall data quality in Salesforce. It encompasses policies, procedures, and controls that ensure data is managed, accessed, and used responsibly across the organization. Challenges in data governance include defining clear ownership of data, establishing data stewardship roles, enforcing compliance with data standards, and maintaining alignment with regulatory requirements.
8. Data Security and Privacy:
Protecting sensitive data from unauthorized access, breaches, and compliance violations is a critical challenge. Salesforce contains valuable customer information and organizational data that must be safeguarded against internal and external threats. Organizations must implement robust data encryption, access controls, audit trails, and regular security assessments to mitigate risks and ensure data security and privacy compliance.
Strategies for Achieving and Maintaining Data Quality
Achieving and maintaining high-quality data is essential for organizations aiming to leverage data-driven insights effectively. This comprehensive process involves several strategic approaches and ongoing practices to ensure that data remains accurate, consistent, reliable, and relevant over time.
1. Data Governance:
Data governance forms the foundation for effective Data quality in Salesforce management. It involves establishing clear policies, procedures, and responsibilities for managing data across the organization. Key aspects include defining data ownership, establishing data standards, and ensuring compliance with regulatory requirements. By instituting robust governance frameworks, organizations can maintain accountability and consistency in data management practices.
2. Data Profiling and Assessment:
Regular data profiling is essential to understand the quality and characteristics of organizational data. This process involves analyzing data to identify inconsistencies, anomalies, and completeness issues. By conducting thorough assessments, organizations can pinpoint areas needing improvement and prioritize Data quality in Salesforce enhancement efforts.
3. Data Cleaning and Enrichment:
Data cleaning and enrichment procedures are crucial for maintaining data accuracy and relevance. This involves processes such as deduplication, normalization, and validation to eliminate errors and inconsistencies. Additionally, data enrichment enhances existing data with additional relevant information, thereby improving its value and utility for analytical purposes.
4. Data Integration:
Seamless data integration is vital for ensuring that data from disparate sources can be combined and analyzed effectively. Using Extract, Transform, Load (ETL) tools and integration platforms, organizations can unify data while preserving its quality and integrity. This enables stakeholders to access consolidated, reliable data for decision-making purposes.
5. Data Quality Metrics:
Defining and monitoring Data quality in Salesforce metrics is essential for assessing and maintaining data integrity. Metrics such as completeness, accuracy, consistency, and timeliness provide quantifiable benchmarks for evaluating data quality levels. Regular monitoring and analysis of these metrics enable organizations to identify trends, detect anomalies, and take corrective actions promptly.
6. Data Validation and Testing:
Implementing rigorous validation and testing processes helps ensure data accuracy and reliability. This involves applying validation rules and conducting systematic tests to verify data integrity across various dimensions. By validating data against predefined criteria, organizations can mitigate risks associated with erroneous data and uphold quality standards.
7. Training and Awareness:
Educating staff about the importance of Data quality in Salesforce and best practices is critical for fostering a data-driven culture. Training programs should emphasize data management principles, data handling protocols, and the impact of high-quality data on organizational outcomes. By promoting awareness and competence among employees, organizations can enhance data stewardship and accountability.
8. Continuous Improvement:
Data quality in Salesforce management is an iterative process that requires continuous improvement and optimization. Organizations should regularly review and refine data management strategies based on feedback, technological advancements, and evolving business needs. Continuous improvement efforts ensure that data quality initiatives remain relevant and effective over time.
9. Data Security and Compliance:
Protecting data security and ensuring compliance with regulatory requirements are integral to maintaining Data quality in Salesforce. Implementing robust security measures, access controls, and data encryption safeguards sensitive information from unauthorized access and breaches. Compliance with data protection regulations also reinforces trust and accountability in data management practices.
10. Stakeholder Engagement:
Engaging stakeholders, including end-users and decision-makers, is essential for aligning Data quality in Salesforce initiatives with organizational objectives. Soliciting feedback, gathering requirements, and addressing stakeholders’ concerns facilitate collaborative efforts in enhancing data quality. By involving stakeholders throughout the data lifecycle, organizations can prioritize data quality improvements that meet user expectations and support strategic goals.
Best Practices for Data Quality Management
Data quality in Salesforce management is essential for organizations seeking to maximize the value of their data assets and make informed decisions. It encompasses a set of best practices aimed at ensuring data accuracy, consistency, completeness, reliability, and timeliness throughout the data lifecycle. Here are the key best practices for effective data quality management:
1. Clear Data Governance:
Data governance provides the framework for managing Data quality in Salesforce effectively. It involves defining policies, procedures, roles, and responsibilities for data management across the organization. Clear governance ensures accountability, transparency, and consistency in data practices, aligning data initiatives with business objectives and regulatory requirements.
2. Define Data Quality Standards:
Establishing Data quality in Salesforce standards is crucial for setting benchmarks and expectations for data integrity. Define specific metrics for completeness, accuracy, consistency, timeliness, and validity tailored to organizational needs. These standards serve as guidelines for evaluating and improving data quality over time.
3. Conduct Data Profiling and Assessment:
Regular data profiling and assessment are essential for understanding the characteristics and quality of organizational data. Data profiling involves analyzing data to identify anomalies, duplicates, and inconsistencies. Assessments help prioritize areas for improvement and guide data cleansing, integration, and enrichment efforts.
4. Implement Data Quality Tools and Technologies:
Utilize Data quality in Salesforce tools and technologies to automate processes such as data cleansing, deduplication, normalization, and validation. These tools streamline data management tasks, enhance accuracy, and reduce manual errors. Choose tools that align with organizational requirements and integrate seamlessly with existing systems.
5. Data Integration and Compatibility:
Effective data integration ensures seamless data flow across systems and applications while maintaining data quality. Implement robust Extract, Transform, Load (ETL) processes or integration platforms to consolidate and unify data from disparate sources. Ensure compatibility and consistency in data formats, structures, and definitions to facilitate accurate analysis and reporting.
6. Establish Data Quality Monitoring and Reporting:
Deploy monitoring mechanisms to track Data quality in Salesforce metrics in real-time or at scheduled intervals. Establish dashboards and reports that provide visibility into data quality levels, trends, and exceptions. Proactively identify issues, anomalies, or deviations from established standards to initiate timely corrective actions.
7. Implement Data Validation and Testing:
Integrate validation rules and conduct systematic testing to verify data accuracy and reliability. Implement data validation checks during data entry, transformation, and integration processes. Perform comprehensive testing to ensure adherence to data quality standards and mitigate risks associated with erroneous data.
8. Foster Data Quality Awareness and Training:
Educate stakeholders, including data stewards, analysts, and end-users, about the importance of Data quality in Salesforce and best practices. Offer training programs to enhance understanding of data management principles, quality standards, and tools. Foster a culture of data stewardship and accountability across the organization to ensure continuous improvement in data quality.
9. Data Security and Compliance:
Adopt robust security measures to protect data integrity, confidentiality, and availability. Implement access controls, encryption, and data masking techniques to safeguard sensitive information from unauthorized access or breaches. Ensure compliance with data protection regulations and industry standards to maintain trust and mitigate legal risks.
10. Continuously Improve Data Quality Processes:
Data quality in Salesforce management is an iterative process that requires ongoing evaluation and improvement. Regularly review data quality metrics, processes, and technologies to identify areas for enhancement. Solicit feedback from stakeholders, monitor industry trends, and incorporate lessons learned to optimize data quality management practices.
Conclusion:
Data quality in Salesforce is crucial for maintaining accurate and reliable customer data, which forms the backbone of effective CRM (Customer Relationship Management). Salesforce provides robust tools and functionalities to manage data quality throughout its lifecycle. Key strategies include defining data governance policies, establishing data quality standards, and leveraging data cleansing and validation tools.
By implementing these practices, organizations can ensure that their Salesforce instance contains clean, consistent, and up-to-date data. This enhances user trust, improves decision-making processes, and supports personalized customer interactions. Salesforce’s data quality features, such as duplicate management, validation rules, and data enrichment capabilities, empower users to maintain high standards of data accuracy and integrity.
Continuous monitoring, training, and integration with external data sources further enhance Salesforce’s effectiveness in maintaining data quality. Ultimately, prioritizing data quality in Salesforce not only optimizes CRM operations but also enhances overall business performance by leveraging reliable data insights.