Mastering Data Quality in Salesforce 2025
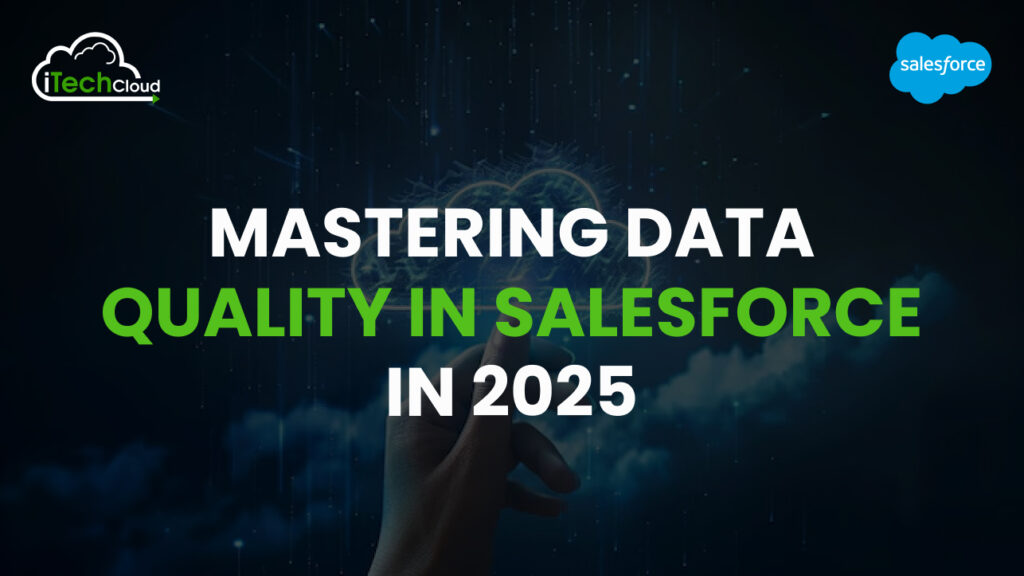
In the rapidly evolving world of customer relationship management (CRM), Salesforce remains a dominant player, empowering businesses to streamline their operations and build lasting relationships with customers. However, the effectiveness of any CRM system, including Salesforce, heavily depends on the quality of data it holds. Poor data quality can lead to inaccurate analytics, ineffective marketing, missed sales opportunities, and decreased customer satisfaction.
As organizations continue to embrace AI-powered automation and personalization at scale in 2025, ensuring high-quality data in Salesforce has become more critical than ever. Here’s a comprehensive guide to mastering data quality in Salesforce this year, exploring the best practices, advanced techniques, and cutting-edge tools that organizations can leverage.
Table of Contents
Why Data Quality is Crucial in 2025
Data quality has a profound impact on business performance. Inaccurate or incomplete data can have the following consequences:
- Poor Customer Experience: Inconsistent or duplicated records lead to miscommunications and missed opportunities.
- Inefficient Operations: Time and resources wasted correcting data errors instead of focusing on value-driven tasks.
- Inaccurate Reporting & Analytics: Faulty data skews reports and decision-making, leading to incorrect business strategies.
- Missed Revenue Opportunities: Poor data can lead to missed cross-sell and up-sell opportunities.
In 2025, with Salesforce integrating AI, predictive analytics, and automation into its ecosystem through Einstein AI and Salesforce Data Cloud, high-quality data becomes a prerequisite for maximizing ROI and customer satisfaction.
Key Dimensions of Data Quality in Salesforce 2025
To maintain high-quality data in Salesforce, organizations should focus on six critical dimensions:
1. Accuracy:
Ensuring that data entered in Salesforce is correct and error-free is essential. Inaccurate data can lead to poor decision-making and reduced customer satisfaction. Implementing validation rules and regular audits helps maintain data accuracy.
2. Completeness:
All essential fields should be filled to avoid incomplete records. Missing data can create gaps in customer information, impacting sales, marketing, and service efforts. Required field enforcement and mandatory data input at key stages can ensure completeness.
3. Consistency:
Data should be consistent across various records and objects. Inconsistencies may occur when data is updated in multiple locations but not synchronized properly. Standardizing data entry processes and automating synchronization between systems can improve consistency.
4. Timeliness:
Up-to-date information is crucial for effective decision-making. Delayed or outdated data can lead to missed opportunities or errors. Regular updates, automated workflows, and scheduled data imports help ensure data remains timely.
5. Uniqueness:
Duplicate records should be avoided to maintain a clean and organized database. Duplicate data can result in inefficiencies and confusion. Using duplicate detection tools and periodic deduplication processes prevents redundancy.
6. Relevance:
Only capturing necessary and meaningful data helps prevent clutter. Irrelevant data can reduce system performance and make it difficult to analyze important information. Defining data collection standards and periodically reviewing data fields ensures relevance.
Challenges in Maintaining Data Quality in Salesforce
Despite Salesforce’s strong infrastructure, maintaining data quality can be difficult due to several challenges:
1. Manual Data Entry Errors:
Human error is one of the most common sources of poor data quality. Incorrect or inconsistent data entry can lead to inaccurate records, making it difficult to maintain reliable information. Regular training and implementing validation rules can help minimize these errors.
2. Duplicate Records:
Merging data from multiple sources often leads to duplicate entries in Salesforce. Duplicate records create confusion and inefficiencies in managing customer information. Using duplicate detection and deduplication tools can help identify and merge duplicate records.
3. Incomplete Data Migration:
Improper or incomplete data migration during Salesforce implementation can result in missing critical information. Data gaps can affect customer insights and overall business processes. Careful data mapping, validation, and post-migration audits are essential to prevent this issue.
4. Lack of Defined Data Governance:
Without established data governance policies, inconsistencies and discrepancies can arise over time. A lack of standardized processes for data entry, updates, and maintenance increases the risk of poor data quality. Implementing strong data governance frameworks ensures consistency and accuracy.
5. Integration with External Systems:
Integrating Salesforce with third-party platforms often introduces inaccuracies due to differences in data formats and synchronization issues. Inconsistent or delayed data updates can compromise data quality. Establishing automated synchronization and regular data audits can mitigate these challenges.
Best Practices for Mastering Data Quality in Salesforce in 2025
1. Implement a Data Governance Framework
Data governance is essential for maintaining data integrity. Establish clear rules and responsibilities that define:
- Data ownership and stewardship.
- Data entry guidelines to maintain consistency.
- Standards for data validation, monitoring, and reporting.
In 2025, companies are adopting AI-powered governance tools that automatically flag inconsistencies and provide recommendations for corrections.
2. Automate Data Validation and Cleansing
Leverage Salesforce’s validation rules, duplicate management rules, and workflow automation to ensure that data entered adheres to set standards. Use AI-based data cleansing tools to automatically identify and correct errors.
3. Data Deduplication Techniques
Duplicate records remain a persistent challenge in Salesforce. Utilize Salesforce’s native Duplicate Management tool to set up matching and merge rules. Advanced AI-powered tools such as Cloudingo, RingLead, and DemandTools can automatically detect and merge duplicates across objects and records.
4. Utilize Salesforce Data Cloud for Real-Time Data Management
Salesforce Data Cloud (formerly Customer Data Platform) empowers organizations to manage and unify customer data from multiple sources. By consolidating fragmented data into a unified customer profile, businesses can eliminate inconsistencies and duplication.
5. Conduct Regular Data Audits and Assessments
Perform regular data quality audits to identify areas of improvement. Set up scheduled reports and dashboards in Salesforce to monitor data quality KPIs such as completeness, accuracy, and duplication rates.
6. Enforce Standardized Data Entry Processes
Design standardized data entry processes with required fields and picklists to ensure consistent data capture. Use Lightning Flow and Process Builder to guide users through data entry, reducing errors and inconsistencies.
7. Enable Real-Time Data Enrichment
Enrich customer records using data from external sources to fill in missing details. AI-powered data enrichment tools such as Clearbit, ZoomInfo, and InsideView automatically populate missing data, enhancing the completeness and relevance of records.
8. Develop User Training and Awareness Programs
Ensure that Salesforce users understand the importance of data quality and follow best practices. Conduct regular training programs, emphasizing the impact of poor data on business outcomes.
Advanced Techniques for Improving Data Quality in Salesforce
Ensuring high data quality in Salesforce is essential for accurate reporting, improved decision-making, and enhanced customer experiences. Poor data quality can lead to missed opportunities, inefficient operations, and reduced trust in data. Below are some advanced techniques to improve and maintain data quality in Salesforce:
1. Data Standardization and Normalization
Standardizing data formats and ensuring uniformity in fields like phone numbers, addresses, and email formats is key. Use validation rules to enforce specific patterns and formats and picklists to limit data entry errors. Implementing standardized naming conventions across objects also helps maintain consistency.
2. Duplicate Management and Prevention
Duplicate records can skew reports and waste resources. Enable duplicate rules and matching rules in Salesforce to automatically detect and merge duplicates. Tools like Duplicate Check or DemandTools can further help automate deduplication processes.
3. Automation with Data Enrichment Tools
Integrate third-party tools such as Clearbit, ZoomInfo, or D&B Hoovers to automatically enrich data with relevant details. Enrichment ensures that records stay up to date by adding missing information and correcting outdated data.
4. Automated Data Validation and Cleansing
Leverage Salesforce Flow and Apex Triggers to automate data validation and perform background checks for anomalies. Regularly schedule data cleansing routines to identify and rectify errors. Automated jobs can detect incomplete or inconsistent records and trigger corrective actions.
5. Custom Error Handling and Alerts
Set up real-time error handling and alert mechanisms to notify relevant teams when data inconsistencies occur. Utilize Process Builder or Flow to create real-time notifications, ensuring that corrective actions are taken promptly.
6. Data Governance and Audit Policies
Establish clear data governance policies, including data ownership, user permissions, and audit procedures. Use field history tracking and audit trails to monitor data changes and enforce accountability.
7. Periodic Data Audits and Cleanup
Conduct regular data audits using Data Loader or Salesforce Inspector to analyze and clean data. Identify redundant or outdated records and archive them as needed. Automated scheduling of audit tasks ensures continuous monitoring.
8. AI-Powered Predictive Data Quality
Salesforce Einstein Analytics to analyze patterns and predict potential data quality issues. Machine learning models can help detect anomalies and suggest corrections before they affect business processes.
Top Tools for Data Quality Management in Salesforce in 2025
In 2025, maintaining high-quality data in Salesforce is essential for accurate reporting, improved customer insights, and efficient decision-making. Several top tools help organizations manage and maintain clean, reliable data:
1. Cloudingo
An AI-powered tool for deduplication and data cleansing. Cloudingo automates the identification and merging of duplicate records, reducing redundancy and ensuring data consistency. Its intuitive interface and advanced AI algorithms make it easy to manage large data sets effectively.
2. DemandTools
Ideal for bulk data processing and cleansing. It allows users to automate complex data management tasks such as deduplication, standardization, and record matching. DemandTools offers powerful features to ensure data integrity and accuracy, making it a trusted solution for Salesforce admins.
3. DataGroomr
Focused on real-time data auditing and deduplication. It leverages machine learning to identify duplicates and inconsistencies, enabling organizations to maintain data accuracy. DataGroomr’s automated processes ensure continuous monitoring and cleaning of Salesforce records.
4. Openprise
A comprehensive solution for end-to-end data orchestration and quality management. Openprise automates data enrichment, deduplication, and governance processes. It ensures that Salesforce data remains clean, standardized, and compliant with business rules.
5. MuleSoft
An API-driven data integration and enrichment platform. MuleSoft facilitates seamless data flow between Salesforce and other systems, ensuring that data is synchronized, enriched, and accurate across all platforms. It helps organizations maintain high data quality by preventing data silos and inconsistencies.
The Future and Trends in Salesforce Data Quality in 2025
In 2025, Salesforce data quality will be driven by advancements in AI, automation, and enhanced data governance practices. Organizations will increasingly prioritize maintaining high-quality data to improve customer experiences, decision-making, and operational efficiency.
1. AI-Powered Data Quality Management
AI and machine learning will play a pivotal role in identifying, cleaning, and enriching data. Automated tools will detect duplicate records, inconsistencies, and anomalies in real time, reducing manual intervention and errors. Predictive models will identify potential data quality issues before they impact business processes.
2. Real-Time Data Validation and Enrichment
Salesforce will offer more advanced real-time data validation capabilities to ensure that data entered into the system meets predefined quality standards. Integration with external data sources will automatically enrich customer profiles, ensuring up-to-date and accurate information.
3. Automated Data Governance and Compliance
Stringent data regulations such as GDPR and CCPA will drive companies to adopt automated data governance frameworks. These frameworks will ensure compliance by enforcing data quality policies, reducing the risk of data breaches, and maintaining data integrity.
4. Hyper-Personalization and Data Accuracy
Salesforce users will leverage high-quality data to drive hyper-personalization. Accurate, well-segmented data will enable targeted marketing, improved sales strategies, and better customer support.
5. Integration of IoT and Big Data
The proliferation of IoT devices and Big Data will introduce vast amounts of data into Salesforce. Advanced data quality tools will be essential to manage, cleanse, and validate this data to derive actionable insights.
6. Self-Service Data Quality Tools
Businesses will empower non-technical users with self-service tools that allow them to assess and maintain data quality independently, promoting a culture of data ownership.
Conclusion:
Maintaining data quality in Salesforce is crucial for improving customer relationships, enhancing reporting accuracy, and driving business growth. Regular audits, data validation rules, and duplicate management help prevent errors and maintain clean records. Standardizing data input processes and encouraging team collaboration ensures consistency. Leveraging AI and automation tools further enhances data quality by reducing manual mistakes.