How to Use Salesforce AutoML for Smarter Decision-Making
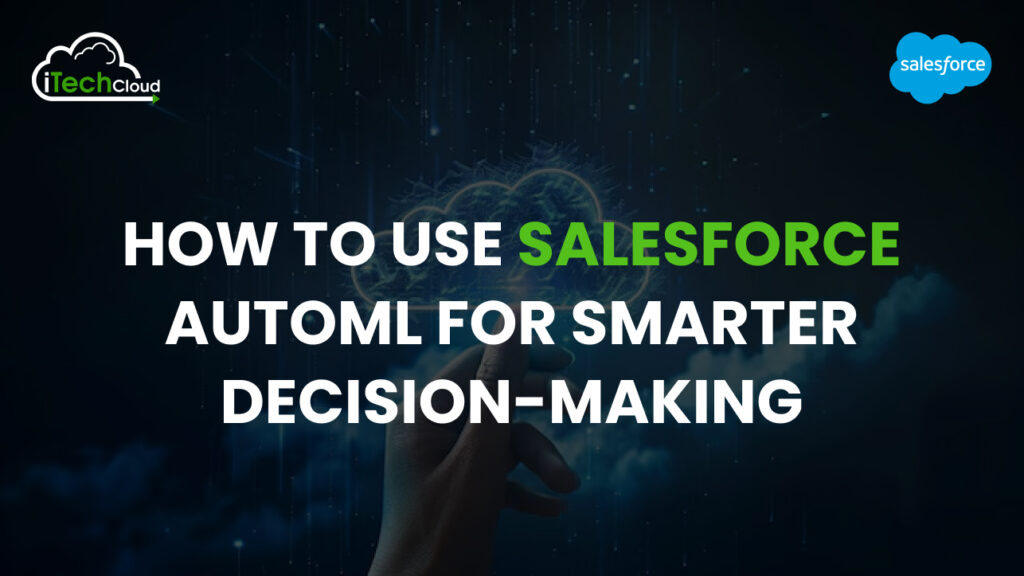
In today’s data-driven world, businesses thrive on making informed decisions. Machine learning (ML) is a powerful tool that enables companies to predict trends, enhance customer experience, and optimize operations. However, implementing ML models requires technical expertise, time, and resources. This is where Salesforce AutoML comes in.
Salesforce AutoML is an automated machine learning solution integrated into Salesforce Einstein. It allows businesses to build, deploy, and manage predictive models without extensive data science knowledge.
This blog will explore how Salesforce AutoML works, its benefits, and how businesses can use it for smarter decision-making.
Table of Contents
What is Salesforce AutoML?
Salesforce AutoML is a feature within Einstein Discovery, a part of the Salesforce Einstein AI platform. It automates the process of training and deploying machine learning models, enabling users to make data-driven decisions without writing complex code.
Key Features of Salesforce AutoML:
- Automated Data Processing: Prepares and cleans data for optimal model performance.
- Model Selection: Identifies the best ML model for a given dataset.
- Explainable AI (XAI): Provides insights into how the model makes predictions.
- Seamless Salesforce Integration: Works natively with Salesforce CRM and cloud services.
- Real-time Predictions: Offers actionable insights within Salesforce dashboards.
How Salesforce AutoML Works
Salesforce AutoML follows a structured process to generate insights from data. Here’s a step-by-step breakdown:
1. Data Preparation
The first step involves collecting and organizing data. Salesforce AutoML automatically cleans and preprocesses data to remove inconsistencies, missing values, and outliers. Users can upload data from Salesforce objects, external sources, or structured CSV files.
2. Model Training & Selection
AutoML applies multiple ML algorithms to find the best-performing model. It evaluates different models based on accuracy, performance, and explainability. The platform then selects the most suitable model for deployment.
3. Model Validation
Once a model is trained, it undergoes rigorous validation to ensure reliability. Salesforce AutoML uses statistical techniques such as cross-validation and A/B testing to measure effectiveness.
4. Model Deployment & Predictions
After validation, the model is deployed within the Salesforce ecosystem. Users can generate predictions in real-time and integrate them into workflows, dashboards, or automated processes.
5. Continuous Improvement
Salesforce AutoML continuously monitors model performance and suggests improvements. Businesses can retrain models as new data becomes available to enhance accuracy and adaptability.
Benefits of Using Salesforce AutoML
1. Improved Decision-Making
AutoML enables businesses to make data-backed decisions with confidence. Whether forecasting sales, identifying churn risks, or optimizing marketing strategies, AutoML provides actionable insights.
2. Cost & Time Efficiency
Traditional machine learning projects require dedicated data scientists, which can be expensive and time-consuming. AutoML automates the process, reducing costs and accelerating deployment.
3. User-Friendly Interface
AutoML is accessible to non-technical users. Salesforce admins, analysts, and marketers can easily leverage AI without advanced ML knowledge.
4. Seamless Salesforce Integration
Since AutoML is built within Salesforce, it integrates effortlessly with CRM data, marketing automation, and analytics tools. Businesses can extract insights without switching platforms.
5. Explainability & Transparency
Salesforce AutoML provides explainable AI (XAI), ensuring users understand how predictions are made and why certain decisions are suggested.
Use Cases of Salesforce AutoML for Smarter Decision-Making
1. Sales Forecasting
AutoML can analyze historical sales data to predict future trends. Sales teams can use these insights to set realistic targets, allocate resources, and optimize strategies.
2. Customer Churn Prediction
AutoML can identify at-risk customers. Businesses can take proactive steps, such as personalized offers, to reduce churn.
3. Lead Scoring & Conversion Optimization
AutoML evaluates lead quality based on interactions, demographics, and engagement. This helps sales teams prioritize high-value leads and increase conversion rates.
4. Marketing Campaign Optimization
Marketers can use AutoML to predict campaign performance, segment audiences, and personalize messaging. This results in better ROI and higher engagement rates.
5. Fraud Detection & Risk Management
AutoML can detect unusual patterns in financial transactions, helping businesses prevent fraud. It can also assess risks associated with loans, insurance claims, or investments.
Best Practices for Using Salesforce AutoML
1. Ensure High-Quality Data
Garbage in, garbage out. The accuracy of AutoML predictions depends on the quality of data provided. Ensure that data is clean, relevant, and up-to-date.
2. Understand Business Goals
Define clear objectives before implementing AutoML. Whether improving sales performance or customer retention, align ML models with business goals.
3. Leverage Explainability Features
Use the explainable AI features to understand model insights. This helps in building trust and making data-driven decisions.
4. Monitor & Update Models Regularly
AutoML models need continuous monitoring to maintain accuracy. Regularly retrain models with new data to improve performance.
5. Integrate Predictions into Workflows
Maximize the impact of AutoML by integrating its predictions into Salesforce workflows, reports, and automated processes.
Conclusion
Salesforce AutoML is a game-changer for businesses looking to harness the power of machine learning without the complexity. By automating the ML pipeline, it empowers companies to make smarter, data-driven decisions effortlessly. From sales forecasting to customer retention, AutoML provides predictive insights that drive growth and efficiency.